AI drug discovery accelerates breakthroughs in medicine
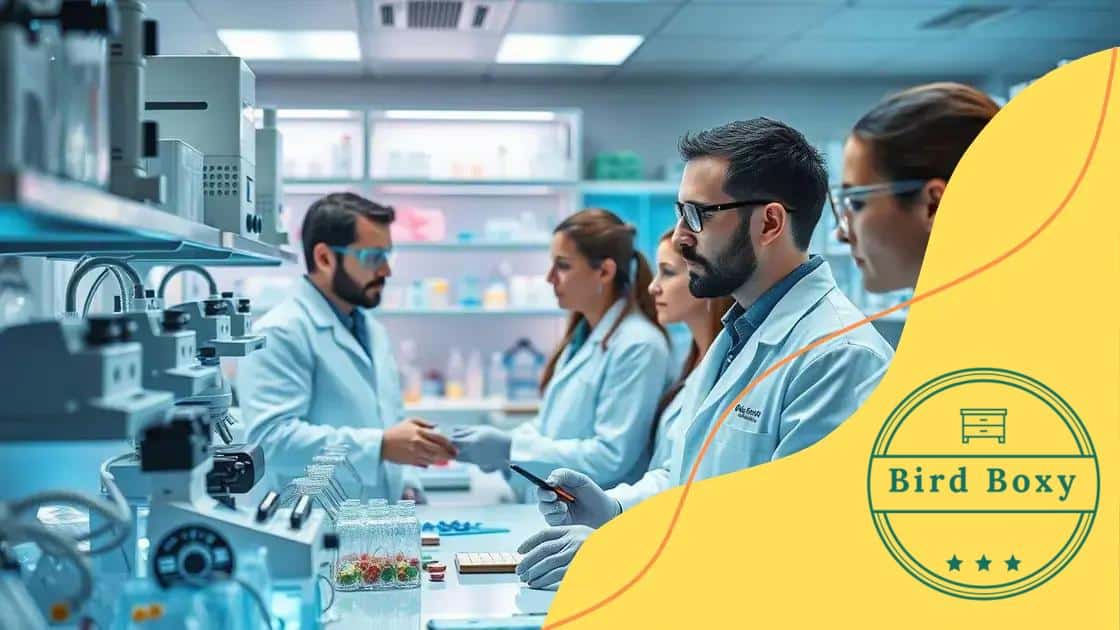
AI drug discovery accelerates the process of identifying new treatments by analyzing large datasets quickly, enhancing the efficiency and accuracy of pharmaceutical research and patient care.
AI drug discovery accelerates the search for innovative treatments, transforming how researchers approach complex medical challenges. Have you ever wondered how technology shapes the future of healthcare? This exploration reveals the exciting intersection of AI and pharmaceutical advancements.
Understanding AI in drug discovery
Understanding AI in drug discovery is crucial for grasping how technology transforms the medical field. Artificial Intelligence plays a key role in quickly analyzing vast amounts of data, helping researchers find new drugs more efficiently.
AI methods can streamline the drug discovery process, making it faster and more cost-effective. By leveraging machine learning techniques, scientists can predict how different compounds will behave in the body, which can greatly reduce the time spent in clinical trials.
Key AI Techniques in Drug Discovery
Some of the most common AI techniques used in this field include:
- Data mining: Extracting valuable information from large datasets.
- Predictive modeling: Forecasting the effectiveness of drugs before they reach the testing phase.
- Natural language processing: Analyzing scientific literature to gather insights.
- Computer vision: Helping researchers analyze images from experiments.
These innovations enable faster identification of potential drug candidates. With tools like virtual screening, AI evaluates compounds against various targets, highlighting the most promising ones to pursue further.
Another important aspect of AI in drug discovery is its ability to identify new uses for existing medications, a process known as drug repurposing. By analyzing data trends, AI systems can suggest alternative therapies that researchers may not have considered, ultimately benefiting patients.
In summary, understanding AI in drug discovery reveals its potential to accelerate the entire process, making it more efficient and effective in finding lifesaving treatments.
How AI enhances research efficiency
How AI enhances research efficiency is an important topic in modern drug discovery. With advancements in technology, researchers are now able to conduct experiments and analyze data much faster than before. AI tools can process large datasets quickly, which allows scientists to make informed decisions without wasting time.
One significant way AI enhances efficiency is through automating repetitive tasks. For example, data entry and preliminary analyses can now be done by AI systems, freeing researchers to focus on more complex problems. This not only speeds up the overall research process but also reduces the chances of human error.
Key AI Applications in Research
Several applications illustrate how AI can improve research efficiency:
- Predictive analytics: AI can forecast outcomes from experiments, allowing researchers to prioritize which experiments to pursue.
- Real-time data analysis: Researchers can receive immediate insights during experiments, helping them adjust their methods on the fly.
- Literature review automation: AI systems can scan and summarize vast amounts of scientific literature, pinpointing relevant studies and data.
- Drug design simulations: AI can simulate how new drugs interact with biological systems, saving time and resources in the lab.
Utilizing AI tools leads to a more structured approach in research. By organizing data and providing insights, AI allows for better collaboration among researchers. Teams can access shared information quickly, helping them innovate and solve problems faster.
As AI continues to evolve, its potential to enhance research efficiency will only increase. Researchers who adopt these technologies can stay ahead in the race to discover new medicines.
Real-world applications of AI in pharmaceuticals
Real-world applications of AI in pharmaceuticals showcase the transformative power of technology in drug development and patient care. AI technologies are not just theoretical; they are actively changing how we approach healthcare challenges worldwide.
AI is being used extensively to enhance the drug discovery process. For instance, it can analyze chemical structures and predict their effectiveness much faster than traditional methods. This leads to earlier identification of potential candidates for clinical trials.
Notable Applications of AI
Some key ways AI is applied within the pharmaceutical industry include:
- Clinical trial optimization: AI assists in patient recruitment by identifying suitable candidates based on medical histories and genetic profiles.
- Personalized medicine: AI algorithms analyze patient data to develop tailored treatment plans, improving outcomes.
- Drug safety monitoring: AI technology enable real-time analysis of adverse drug reactions by mining data from electronic health records.
- Supply chain management: AI helps forecast demand and optimize inventory levels, ensuring timely delivery of medications.
Furthermore, AI is revolutionizing healthcare analytics. By using big data, AI provides valuable insights into disease patterns, treatment efficacy, and patient preferences. This allows pharmaceutical companies to make strategic decisions that align with patient needs.
As these applications continue to evolve, the integration of AI in pharmaceuticals is expected to advance, fostering innovation and improving patient outcomes across the globe.
Challenges faced in AI drug discovery
Challenges faced in AI drug discovery highlight the complexities that come with integrating technology into pharmaceutical research. While AI offers exciting promises, various obstacles still need to be addressed.
One major challenge is the quality of data. AI systems rely on large datasets to learn and make predictions. If the data is inaccurate or biased, the outcomes can be flawed, leading to ineffective drug candidates. Ensuring that datasets are comprehensive and representative of diverse populations is crucial.
Key Challenges in AI Drug Discovery
Several specific challenges continue to affect AI in drug discovery:
- Regulatory hurdles: Navigating the complex regulations and approval processes can be slow and cumbersome for AI-driven approaches.
- Integration with existing systems: Pharmaceutical companies often use legacy systems that are not compatible with new AI technologies, complicating implementation.
- Need for interdisciplinary knowledge: Successfully applying AI requires collaboration among data scientists, medicinal chemists, and healthcare professionals to ensure that technology meets real-world needs.
- Ethical concerns: Using AI in healthcare raises ethical questions, particularly in regards to patient privacy and consent.
Training AI models is another intricate task. These models require extensive tuning and validation to provide reliable results. The need for continuous updates and retraining can increase costs and timelines for drug development.
Despite these challenges, the potential for AI to revolutionize drug discovery remains strong. With ongoing research and collaboration among experts, many of these issues can be addressed, paving the way for smoother integration and more effective treatments in the future.
The future of AI in medical research
The future of AI in medical research is bright and full of possibilities. As technology continues to evolve, AI is expected to play an even larger role in the way we understand health and disease.
One promising area is the use of AI for predictive analytics. By analyzing patient data, AI can help identify potential health issues before they become serious. This proactive approach can lead to earlier interventions and better patient outcomes.
Emerging Trends in AI
Several trends are shaping the future of AI in medical research:
- Genomics and personalized medicine: AI is making it easier to analyze genetic information, allowing for tailored treatments based on individual profiles.
- Enhanced diagnostics: AI-powered tools are improving diagnostic accuracy by analyzing medical images and lab results faster and more reliably.
- Drug repurposing: AI can help identify new uses for existing drugs, speeding up the process of finding effective therapies for various conditions.
- Remote health monitoring: With AI, remote patient monitoring can become more efficient, helping healthcare providers to track patients’ health in real-time from afar.
Collaboration among researchers is also expected to increase. AI can facilitate partnerships by providing platforms for data sharing and collective analysis. This collaboration can lead to groundbreaking discoveries and a more holistic understanding of medical challenges.
As AI continues to advance, ethical considerations will also rise to the forefront. Questions regarding patient privacy and data security will need to be addressed to ensure trust in AI systems.
FAQ – Frequently Asked Questions about AI in Medical Research
How does AI improve drug discovery?
AI analyzes vast amounts of data quickly, helping researchers identify potential drug candidates faster and more efficiently.
What are the main challenges of using AI in pharmaceuticals?
Key challenges include data quality issues, regulatory hurdles, and the need for interdisciplinary collaboration.
Can AI impact patient care?
Yes, AI can enhance patient care by personalizing treatments and improving diagnostic accuracy.
What is the future of AI in medical research?
The future includes advancements in predictive analytics, personalized medicine, and better collaboration among researchers.